import os
import logging
import numpy as np
import weka.core.jvm as jvm
from weka.associations import Associator
from weka.core.converters import Loader
jvm.start(logging_level=logging.ERROR)
if not os.getenv(
"NBGRADER_EXECUTION"
):
%load_ext jupyter_ai
%ai update chatgpt dive:chat
# %ai update chatgpt dive-azure:gpt4o
Association Rule Mining using Weka¶
We will conduct the market-basket analysis on the supermarket dataset in Weka.
Transaction data¶
Each instance of the dataset is a transaction, i.e., a customer’s purchase of items in a supermarket. The dataset can be represented as follows:
Using the Explorer interface, load the supermarket.arff
dataset in Weka.
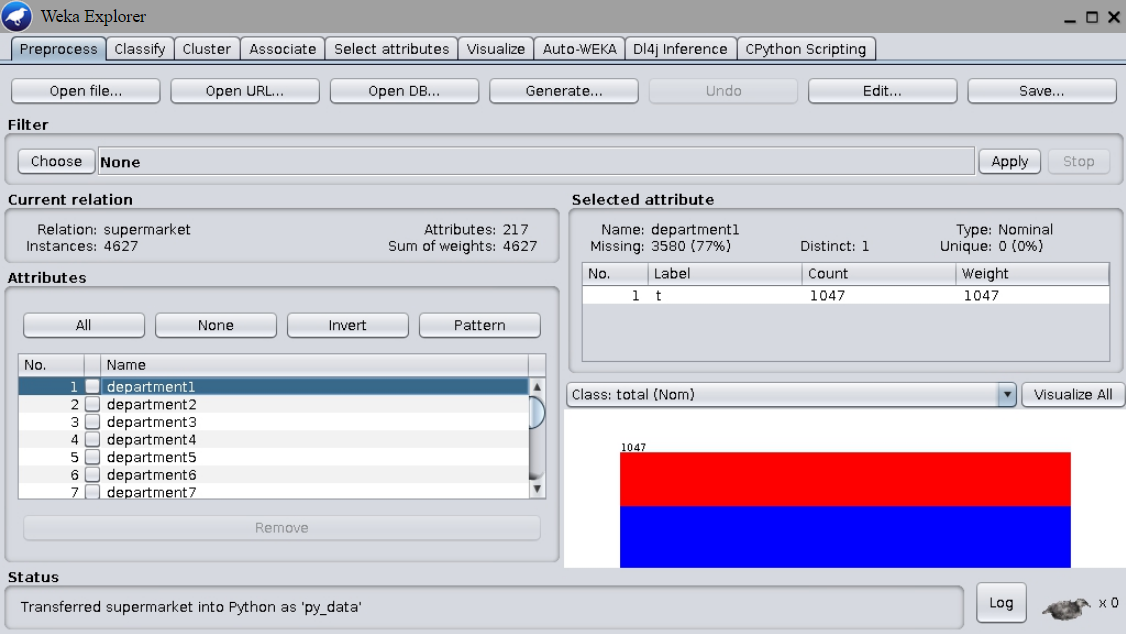
Note that most attribute contains only one possible value, namely t
. Click the button Edit...
to open the data editor. Observe that most attributes have missing values:
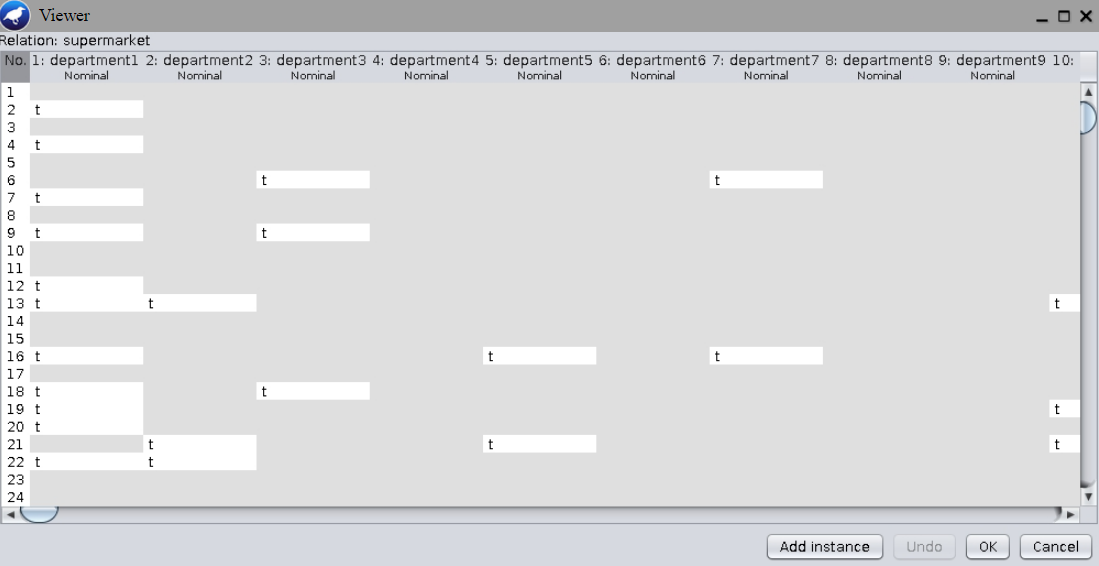
In supermarket.arff
:
- Each attribute specified by
@attribute
can be a product category, a department, or a product with one possible valuet
:
...
@attribute 'grocery misc' { t}
@attribute 'department11' { t}
@attribute 'baby needs' { t}
@attribute 'bread and cake' { t}
...
- The last attribute
'total'
has two possible values{low, high}
:
@attribute 'total' { low, high} % low < 100
To understand the dataset further:
- Select the
Associate
tab. By default,Apriori
is chosen as theAssociator
. - Open the
GenericObjectEditor
and check for a parameter calledtreatZeroAsMissing
. Hover the mouse pointer over the parameter to see more details. - Run the Apriori algorithm with different choices of the parameter
treatZeroAsMissing
. Observe the difference in the generated rules.
YOUR ANSWER HERE
%%ai chatgpt -f text
What is the benefit of `treatZeroAsMissing` in Weka's Apriori Associator?
Association rule¶
An association rule for market-basket analysis is defined as follows:
We will use python-weka-wrapper3
for illustration. To load the dataset:
loader = Loader(classname="weka.core.converters.ArffLoader")
weka_data_path = (
"https://raw.githubusercontent.com/Waikato/weka-3.8/master/wekadocs/data/"
)
dataset = loader.load_url(
weka_data_path + "supermarket.arff"
) # use load_file to load from file instead
To apply the apriori algorithm with the default settings:
from weka.associations import Associator
apriori = Associator(classname="weka.associations.Apriori")
apriori.build_associations(dataset)
apriori
YOUR ANSWER HERE
To retrieve the rules as a list, and print the first rule:
rules = list(apriori.association_rules())
rules[0]
To obtain the set (in premise) and (in consequence):
rules[0].premise, rules[0].consequence
premise_support = rules[0].premise_support
total_support = rules[0].total_support
The apriori algorithm returns rules with large enough support:
For the first rule, the number 723 at the end of the rule corresponds to the total support count .
# YOUR CODE HERE
raise NotImplementedError
support
<conf:(0.92)> lift:(1.27) lev:(0.03) conv:(3.35)
printed after the first rule indicates that
- confidence is used for ranking the rules and
- the rule has a confidence of 0.92.
By default, the rules are ranked by confidence, which is defined as follows:
In python-weka-wrapper3
, we can print different metrics as follows:
for n, v in zip(rules[0].metric_names, rules[0].metric_values):
print(f"{n}: {v:.3g}")
# YOUR CODE HERE
raise NotImplementedError
premise_support
Lift is another rule quality measure defined as follows:
# YOUR CODE HERE
raise NotImplementedError
lift
YOUR ANSWER HERE
YOUR ANSWER HERE
%%ai chatgpt -f text
In association rule mining, what are the pros and cons of ranking the rules
according to lift instead of confidence?